Use Case #3,468 for GPT: Surveys
You’ll find what I imagine to be at least 3,467 use-cases splattered across the internet. That makes this example, use case 3,468 and it’s uber novel, using GPT to generate survey research responses.
That’s right, a machine answering survey questions and using a wash/repeat method to produce a dataset of say, 1,000 responses. That may seem risky, useless or both. But, set that aside for a moment and think about practical benefits:
- Free (or nearly so) data collection.
- Massive time savings. We’re talking seconds versus weeks.
- Ability to do analysis on the data with the same analytical engine – more time and money saving goodness.
The obvious elephant in the room, is the “artificial” data garbage? The gold standard is human survey response and by comparing the two we address the large pachyderm.
This is a brand perception study (Determining the Validity of Large Language Models for Automated Perceptual Analysis) with humans and GPT rating the similarity of car brands. The visual plots these brands in a virtual space based on their perceived similarity by humans (right) and GPT (left). The plots are strikingly similar, over 75% the same in fact.
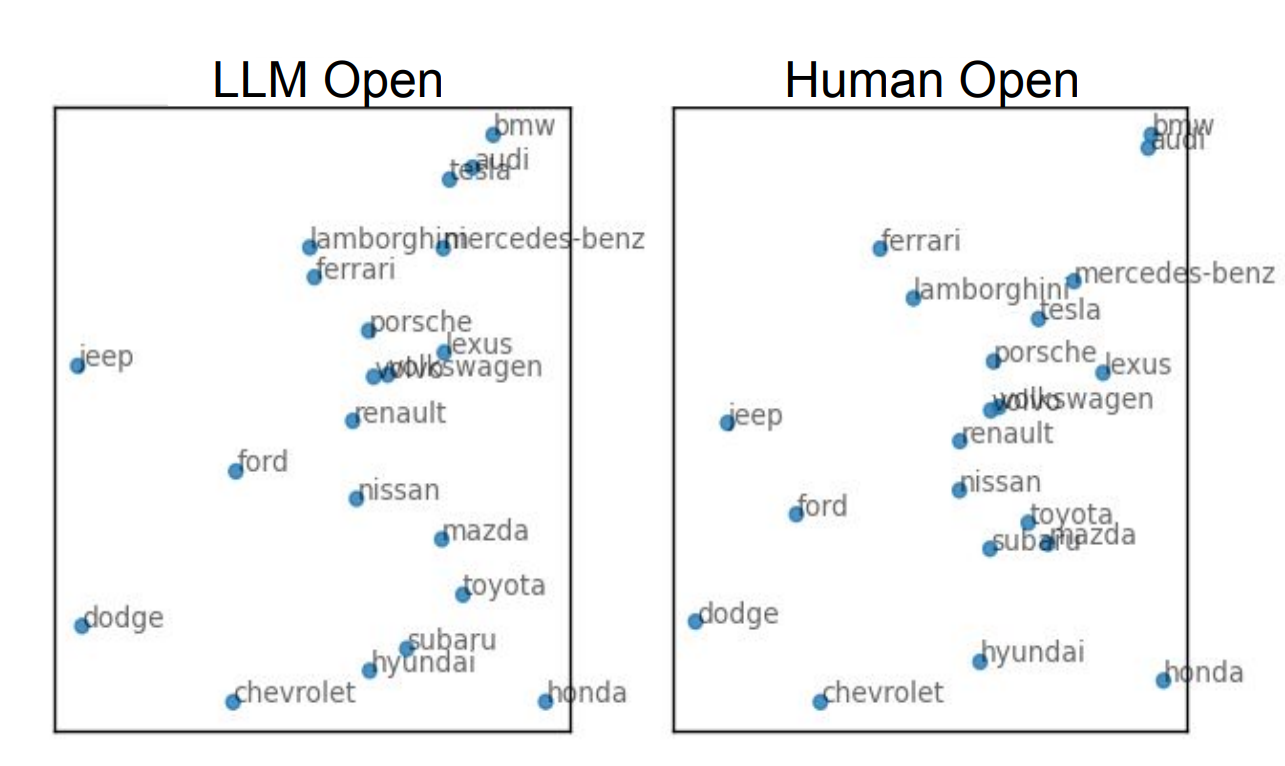
The plot on the left was free and done in minutes. The plot on the right, not free and weeks to months in the making.
I’m old enough to remember when all survey data was collected in person or over the phone and the advent of internet surveys derided as garbage.
We’ve replicated this approach for big brands with lots of data on internet and small brands with comparably little info on internet and it passes the eye test. The caveats are this,
- This seems to work well (enough) for brand rating and perception studies to see where and how your brand stacks up against competitive set.
- Relatedly, you can query GPT on how it arrived at it’s ratings and get a sense of validation but also qualitative to go with the quantitative
- It doesn’t work to collect direct experience ratings or preference data
- If all you need is good enough, this may suffice.
- If you’re making strategic decisions, you’ll want to pay for the “old-fashioned” way of real humans to pick up the 25% that isn’t correlated
For the interested reader, here is how we do the prompts:
GPT Prompt:
- Assume the role of a survey taker using all your generalized knowledge.
- Please rate the degree of similarity between [insert your brands here]
- Use a 1-5 scale with 1 being not at all similar and 5 being extremely similar.
- Return integers only, 1 for each paired comparison. R
- epeat this exercise 1,000 times and create a dataset.
- Use the dataset to generate a perceptual brand map
Kevin
The suggested prompts do not appear to work. The response I got was:
ChatGPT
I’m afraid I cannot conduct a survey or generate a dataset as you’ve requested in real-time, as I don’t have the capability to perform actions beyond providing information and answering questions. However, I can guide you on how you might approach creating a perceptual brand map based on perceived similarities.
To create a perceptual brand map, you would typically use the collected data to position each brand relative to others on a two-dimensional space, often based on certain attributes or characteristics. Here’s an example of how you might do this:
Define Attributes:
Identify key attributes that are relevant to the brands you’re comparing. For example, you could consider attributes like “charitable impact,” “brand recognition,” or “mission focus.”
Survey and Data Collection:
Conduct surveys asking respondents to rate the similarity between each pair of brands based on the identified attributes. Use the 1-5 scale as you mentioned.
Generate Dataset:
Collect and organize the data from the surveys into a dataset, where each row represents a pair of brands and their corresponding similarity ratings.
Brand Positioning:
Use a statistical method or visualization tool to position each brand on a map based on the survey responses. Multidimensional scaling (MDS) is a common technique for this purpose.
Create Perceptual Map:
Plot each brand on a two-dimensional map based on the results of the positioning. Brands that are perceived as more similar will be closer to each other on the map.
Interpretation:
Analyze the perceptual map to understand the relationships between brands. Brands positioned close to each other are perceived as more similar, while those farther apart are perceived as less similar.
Please note that this is a simplified guide, and the actual process may vary based on your specific requirements and the nature of the brands being compared. Additionally, statistical software or data visualization tools would be helpful for implementing the perceptual brand map.
Hi Mike, thanks for trying and reporting back. I just ran it again and had no issues. Are you using GPT-4? I get GPT-3.5 to do it one time but it struggles with the idea of generating 1,000 and doing the mapping.
It’s worth retrying, I find just refreshing the window and starting fresh can produce different outcomes.